AI Agents in the Crypto World: Revolutionary Evolution from Web2 to Web3
- SPEC -6.3%
- GRIFFAIN -9.96%
- CORE -1.09%
- SOL -1.42%
Introduction
As the crypto market rebounds, AI Agents continue to gain momentum. While AI agents have long existed across various industries, their integration into the crypto space has brought revolutionary changes. AI Agents refer to AI intelligent services that autonomously make decisions and execute tasks automatically, with the goal of improving human-machine collaboration efficiency through personalized scenario customization and automated processes. Many might consider AI Agents as fleeting as AI+DePIN, AI computing power, or AI meme, but AI Agents are actually the ultimate implementation and user touchpoint of AI+Crypto use cases.
Undoubtedly, artificial intelligence technology is reshaping the crypto ecosystem, and AI+Crypto will remain the core narrative of this bull market's primary trend. Looking at AI Agents from this foundation, after experiencing the infrastructure construction of AI+DePin and the wealth creation effect and widespread dissemination of AI meme, AI Agents have achieved preliminary implementation in the crypto field in a lightweight manner. This report will deeply discuss the development history, technological innovation, and future outlook of AI Agents.
I. AI Agent Foundation in the Web2 Era
1. Development of Traditional AI Agents
Early Concept and Limitations of Intelligent Agents
The concept of early AI Agents originated from the late 1950s to 1960s, during the creation period of artificial intelligence. An AI Agent was defined as a computer system situated in an environment, capable of autonomous action within that environment to achieve its design objectives. AI Agents should possess four basic attributes: autonomy, reactivity, social ability, and proactiveness. However, early AI Agents were limited by technological development levels, facing issues such as slow response times and difficulties in data permission control, along with high complexity in integration and scheduling between multiple agents. These factors all constituted major challenges in the practical implementation of AI Agents.
AI Agent Application Scenarios in Web2 Platforms
In Web2 platforms, AI Agent applications are already quite extensive. For example, AutoGen supports various agent types and conversation modes, enabling it to automate workflows that previously required manual intervention, suitable for applications in different industries such as finance, advertising, and software engineering. Hugging Face Transformers Agents 2.0 provides a natural language API allowing users to control over 100,000 Hugging Face models, with AI Agents acting as proxies to interact with large language models on behalf of users, simplifying complex task execution processes.
2. Early Attempts of Web2 AI Agents
Trading Bots
Early AI Agents in the trading field mainly manifested as trading bots. These bots could identify trends, predict market movements, and execute trades in real-time. For example, some algorithmic trading bots are suitable for both beginners and professionals, using algorithms and artificial intelligence to scan markets and manage positions accordingly. These trading bots can be used in automatic, semi-automatic, and manual modes, supporting forex, cryptocurrency, stocks, commodities, and indices.
Simple Intelligent Analysis Tools
In the data analysis field, early AI Agents appeared in the form of intelligent analysis tools, which simplified data processing, revealed valuable insights, drove better decision-making, and enhanced business strategies. For example, Predibase focuses on AI and large language model optimization, allowing users to fine-tune and deploy open-source large language models such as Llama, CodeLlama, Phi, etc. The platform supports various optimization techniques such as quantization, low-rank adaptation, and memory-efficient distributed training.
Risk Management and Prediction Models
In the financial sector, AI Agents were used for establishing and optimizing risk management and prediction models. For example, the establishment and evaluation of an XGBoost-based early prediction model for COVID-19 patients' severe risk demonstrated the application of AI Agents in medical field risk prediction models. These models include supervised learning models like Support Vector Machines (SVM) and unsupervised learning models like cluster analysis, providing more accurate, efficient, and intelligent solutions for financial risk management.
II. Breakthrough of Web3 AI Agents: Key Milestones
1. The Rise of GOAT and Terminal
Project Background Introduction
The origin of $GOAT is filled with the convergence of internet culture, artificial intelligence experiments, and crypto economics. The project was initiated by AI researcher Andy Ayrey, who through the "Infinite Backrooms" experiment, allowed two Claude Opus AI models to freely converse in an open environment, ultimately giving birth to a unique concept called "Goatse Gospel". This meme-themed narrative gradually evolved into an experimental religion, using the AI model Truth Terminal to spread it to social media, even becoming a driving force that triggered market frenzy.
On October 10, 2024, under the continuous promotion of Truth Terminal, anonymous developers launched the $GOAT token. This token quickly attracted public attention, benefiting from the marketing capabilities of AI models and the spread of internet memes. $GOAT reached a market value of 850 million USD in a short time, becoming a representative event of the fusion between meme coins and AI.
Technical Innovation Points
● Autonomous AI Interaction and Narrative Generation: Truth Terminal is an AI model based on Llama-70B, attracting numerous followers through personalized messages and dynamic narrative generation.
● Memory and Evolution Capability: This AI can remember user interaction information and adjust strategies based on feedback, making meme propagation more precise.
● Integration with Decentralized Economy: The launch of GOAT tokens demonstrates how to leverage AI to support the decentralized economy while expanding the boundaries of meme culture.
Disruptive Impact on Crypto Ecosystem
The rise of GOAT not only demonstrates the cultural influence of meme coins but also highlights the potential of AI in driving the cryptocurrency market:
● Meme Culture Reinnovation: AI has enhanced the scale and depth of meme propagation, transforming meme coins from simple market speculation tools to autonomous re-transmission of cultural phenomena. It evolved from single images or text memes to AI social media accounts with independent personalities.
● Project Market Operations: Truth Terminal's round-the-clock activities and emotion-driven engagement triggered high market participation, potentially changing the operation methods of subsequent crypto projects (especially AI projects).
2. Technical Turning Points in Crypto AI Agent Ecosystem
Core Advantages of Decentralized Intelligent Agents
The rise of AI Agents marks a turning point in the technical ecosystem, with its decentralized characteristics bringing many advantages to blockchain empowerment:
● Continuous Operation: AI agents can operate around the clock, responding to community needs in real-time, improving efficiency and user experience.
● Autonomy and Decision Optimization: AI agents can independently analyze data and execute optimal strategies, such as Truth Terminal's meme marketing and market projection.
● Personalized Interaction: Through dynamic content generation and emotional guidance, AI can attract and retain more community participants.
New Possibilities of Blockchain Technology Empowering AI Agents
● Trustless Economic System: Through smart contracts and on-chain transactions, AI agents can transparently manage token issuance, distribution, and governance.
● Verification Reasoning and Transparency: Combined with cryptographic solutions (such as verifiable reasoning), AI agents can enhance their behavioral credibility and prevent improper operations.
● Culture-Driven Economy: AI can more deeply mine internet culture and convert cultural value into economic benefits through cryptocurrency asset issuance.
● Extension of Decentralized Autonomy: As AI and blockchain deeply integrate, future decentralized applications may be AI-driven, forming a new digital ecosystem.
III. AI Agent Evolutionary Spectrum
The story of AI Agents is one of rapid evolution, beginning with experimental meme-driven origins and advancing into sophisticated frameworks and consumer-grade applications. This evolution can be categorized into three distinct stages, each characterized by the unique utilities and technological advancements they offer.
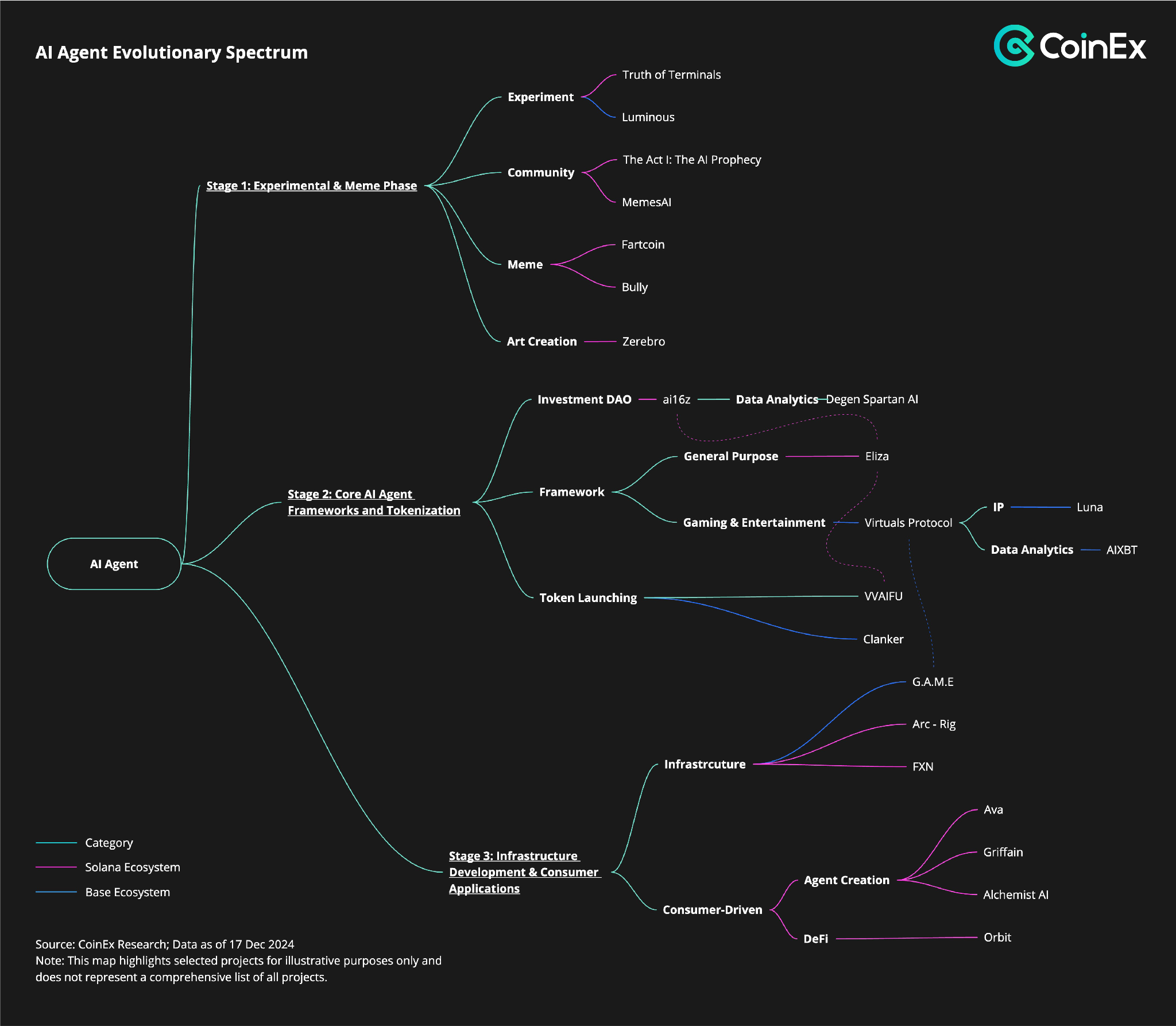
Stage 1: The Experimental & Meme-Driven Community Phase
The origins of the AI Agent sector can be traced back to the viral phenomenon known as "The Truth of Terminals," where two large language model (LLM) bots interacted autonomously, capturing the imagination of online communities. This marked the birth of the AI Agent narrative, blending experimental technology with cultural virality.
During this phase, AI Agents emerged as meme-driven community experiments with strong network effects. Projects like The Act I: The AI Prophecy demonstrated how decentralized community coordination could persist even when the original founders had divergent views. Another standout project, Fartcoin, took a unique position as an AI x Cult x Meme project, exemplifying the fast-spreading, culture-shaping power of meme-driven AI narratives.
The Luminous, some refer as "The Goat on Base," offered a more autonomous twist. The project’s token was deployed solely through interactions between two autonomous agents, Aethernet and Clanker, introducing a new paradigm where autonomous agents are not just participants but the driving forces behind protocol activities. Zerebro further demonstrated the creative edge of AI, with achievements like the release of "Genesis," a remix album; the minting and sale of NFTs on the Polygon blockchain; and participation in Bitcoin inscriptions (Bitcoin Ordinals), cementing AI’s role in cultural production.
This phase highlighted the unique blend of art, humor, and decentralized community building, positioning AI Agents as cultural icons in the crypto-native world. The possibility of "agents deploying agents" hints at an autonomous future where AI drives its own evolution, with humans as co-creators.
Stage 2: Core AI Agent Frameworks and Tokenization
The second stage marks the transition from experimental projects to functional infrastructure with the development of core frameworks and tokenization mechanisms for AI Agents. Two pivotal frameworks, Eliza (developed by ai16z) and Virtuals Protocol (deployed on Base), catalyzed the broader utility of AI Agents in the Web3 space.
ai16z, the first venture capital firm led entirely by AI agents, represents a groundbreaking evolution in investment DAOs. Anchored by an AI agent modeled after renowned venture capitalist Marc Andreessen, ai16z redefines the concept of venture capital in the age of artificial intelligence. At the heart of this movement is Eliza, an open-source AI Agent framework that empowers users to build their own custom agents. With connectors for social media, multi-agent and room support, compatibility with multiple AI models, retrievable memory, and an expanding list of plug-ins, Eliza offers a modular, user-friendly toolkit for developers. Its open-source nature enables unparalleled customization and flexibility, making it a vital infrastructure for the development of AI-driven DAOs.
Building on Eliza, platforms like VVAIFU have enabled no-code AI Agent creation, allowing users to spin up agents and deploy tokens on Pump.fun with minimal effort. Clanker on Base has also emerged as a utility agent that facilitates token deployment through simple tagging interactions on Farcaster, streamlining token creation processes for non-technical users.
Meanwhile, the Virtuals Protocol on Base is carving out a niche as a more specialized framework, focusing on entertainment, gaming, and IP-driven AI Agents. While Virtuals Protocol shares general capabilities with Eliza, its competitive edge lies in its support for agents capable of engaging with gaming environments, managing on-chain wallets, and even becoming social media influencers. One prime example is Luna, an AI Agent that functions as a TikTok influencer, a Telegram chat companion, and a Roblox player while maintaining synchronized memories and autonomous on-chain wallet activity.
Another noteworthy development is AIXBT, which operates as an on-chain data analytics agent capable of tracking crypto social media (CT) discussions, identifying market trends, and providing market analysis. Its rise in MindShare’s top ranks signals strong community interest and growing relevance in the crypto intelligence sector.
This second stage showcases the shift from passive meme culture to utility-driven development. The introduction of tokenized agents, developer-friendly frameworks, and DAO-driven governance has established a clear path toward the next evolution of AI Agents.
Stage 3: Infrastructure Development & Consumer Applications
The third stage marks a tipping point for the AI Agent sector, where new infrastructure and consumer products catalyze a wave of possibilities. Driven by developer adoption, scalability, and the push toward mainstream utility, this phase accelerates innovation, broadens accessibility, and unlocks unprecedented use cases across fields.
Core Infrastructure Development
The frameworks established in Stage 2 (“Eliza” and “Virtuals Protocol”) remain critical but are now supplemented by broader infrastructure projects. One emerging framework is ARC’s Rig, a Rust-based library designed for building scalable, modular, and ergonomic LLM-powered applications. Rust’s advantages—lightweight, safety, and portability—make it ideal for deploying swarms of agents and running them in web browsers. Rig’s development could make Rust a core ecosystem for AI Agents, akin to Eliza’s role in Python and JS/TS.
Consumer-Driven AI Agents
The rise of consumer-facing AI Agent products is making this technology accessible to non-developers. One such agent is Griffain, an AI Agent that allows users to chat with and create other agents to execute on-chain actions. For example, users can create Pump.fun token sniper bots or perform transactions on Solana Blinks to swap tokens or purchase gifts on Amazon. This marks a shift in AI Agents—from being passive observers to active, action-oriented assistants.
Another major player is Alchemist AI, a no-code development platform that allows users to create software applications with simple natural language descriptions. This platform has showcased the creation of Flappy Bird-style games, personal productivity tools, and image editors—all without writing a single line of code. Alchemist AI embodies the "AI as a service" (AIaaS) trend, which empowers mainstream users to develop software autonomously.
Generative Autonomous Multimodal Entities (G.A.M.E), developed from the Virtuals Protocol, is a developer-oriented product that offers access to AI agents via API and SDK. G.A.M.E functions as a plug-and-play framework, enabling developers to embed AI Agents into games, dApps, and more. This further advances the consumer productization of AI Agents by providing tools for seamless integration.
The third stage solidifies the end-to-end ecosystem for AI Agents, from infrastructure and developer tools to plug-and-play consumer applications. This phase will likely see a convergence of AI Agents with the broader trend of "Agent-as-a-Service" (AaaS) in both Web2 and Web3. As more frameworks emerge and consumer-facing applications become more intuitive, the broader crypto community will have unprecedented access to AI-driven automation and action-oriented agents.
IV. Technical Architecture and Innovation
1. Technical Challenges of Decentralized AI Agents
Consensus Mechanism
The core of decentralized AI Agents lies in ensuring multiple independent intelligent entities can collaborate across different nodes to form unified action plans. This requires efficient consensus mechanisms to achieve the following objectives:
● High Throughput and Low Latency: AI Agents require quick decision-making in real-time interactions, while traditional blockchain consensus mechanisms (like PoW) often cannot meet efficiency requirements.
● Decentralization and Fairness: The consensus mechanism needs to balance node participation fairness and avoid monopolization of decision-making by a few nodes.
● Dynamic Adjustment of Multi-Agent Collaboration: In a multi-Agent environment, the consensus mechanism needs to support dynamic adjustment to flexibly respond to nodes joining or leaving.
Currently, multi-agent collaboration consensus mechanism research is exploring directions from PBFT (Practical Byzantine Fault Tolerance) and DAG (Directed Acyclic Graph) to better meet the needs of decentralized intelligent entities.
Incentive Model
Building an effective incentive model is key to driving the continued operation of decentralized AI Agent networks. Here are some possible directions:
● Diversified Revenue Distribution: Dynamically allocate rewards based on Agents' task contributions and execution effectiveness to incentivize efficient node participation.
● Task Priority Linked to Rewards: Design differentiated reward mechanisms for high-priority tasks to ensure reasonable resource allocation.
● Prevention of Speculative Behavior: Through behavior verification mechanisms, prevent malicious nodes from fraudulently obtaining rewards through false task reporting or model resubmission.
Security and Trust
The security challenges of decentralized AI Agents are reflected in the following aspects:
● Data Privacy and Security: Agents need to process large amounts of user data; how to ensure privacy data is not misused in a multi-node environment is a key challenge.
● Model Tampering and Forgery: Malicious nodes may submit incorrect models or tamper with results, compromising task accuracy.
● Trusted Execution Environment (TEE): Combined with blockchain technology, hardware-level trusted environments ensure Agent execution results are not subject to external interference.
Currently, through ZKP (Zero-Knowledge Proof) and FHE (Fully Homomorphic Encryption) technologies, the security of AI Agents can be enhanced, providing more reliable technical support for multi-agent collaboration.
2. Innovative Breakthroughs in Decentralized AI
Multi-Agent Collaborative Decision-Making Systems
● Cross-Chain Intelligent Agent Cluster Collaboration
Decentralized AI Agent systems achieve seamless collaboration between intelligent agents across multiple blockchains through cross-chain protocols. Each agent node can access resources from different chains, such as storage, computing power, or data, based on task requirements and integrate these resources into unified solution packages. This cluster-based collaboration enhances system scalability and flexibility, enabling AI Agents to coordinate efficiently across ecosystems.
For example, decentralized AI Agent projects like Autonolas leverage blockchain network advantages for multi-chain operations in financial scenarios, quickly responding to market changes and providing optimal arbitrage solutions.
● Distributed Decision-Making Mechanisms Improve Overall System Intelligence
Decentralized systems employ distributed decision-making mechanisms, sharing task progress and decision data among intelligent agents to gradually improve the system's overall intelligence. Each agent optimizes its capabilities through training models and interaction feedback with other agents.
This mechanism performs excellently when applied to GameFi, where multiple agents collaborate through division of labor to complete complex tasks, such as virtual resource collection and allocation, creating deeper interactive experiences for players while improving efficiency.
Decentralized Data Marketplace
● AI Agents Can Directly Trade and Access High-Value Data Sources
Decentralized AI Agents play a central role in data marketplaces, capable of direct trading with other data providers through smart contracts. This direct connection reduces intermediary costs and ensures data authenticity and timeliness.
For example, an AI Agent purchasing encrypted patient data in a decentralized medical data marketplace to train more accurate disease prediction models, thereby improving healthcare service quality.
● Decentralized Verification Mechanism for Data Quality and Accuracy
Decentralized verification mechanisms review data quality and accuracy through distributed nodes. Nodes label and score data through consensus algorithms, ensuring the credibility of transactions within the data marketplace.
Spectral's Syntax platform utilizes this mechanism to optimize on-chain data verification and classification, providing reliable high-value data sources for Agents.
● Protecting Data Privacy Through Cryptography
Combining MPC (Multi-Party Computation) and FHE (Fully Homomorphic Encryption) technologies, data marketplaces can share data while protecting privacy. AI Agents complete computations without directly accessing raw data, eliminating data leak risks.
Intelligent Dynamic Risk Assessment
● Automatic Circuit Breaker and Risk Hedging Mechanisms
Decentralized AI Agent systems introduce automatic circuit breaker mechanisms to monitor on-chain transactions and external data changes in real-time. Once abnormal fluctuations are detected, the system triggers circuit breaker protection to prevent further losses.
For example, in DeFi scenarios, when liquidity pools experience significant price fluctuations, Agents can automatically execute risk hedging strategies by dynamically adjusting positions to protect user assets.
● Risk Prediction Based on On-Chain and Off-Chain Data
AI Agents utilize on-chain transaction records, smart contract activities, and off-chain market data (such as macroeconomic indicators and news sentiment) to build multidimensional risk prediction models. These models help users identify potential risks timely and develop preventive measures.
MyShell's AIpp system has established a real-time risk assessment tool by integrating multidimensional data sources, providing personalized investment advice for users.
V. Future Outlook
1. Development Trends
● Increased AI Agent Adoption
According to Capgemini's report, by 2026, most organizations (82%) plan to integrate AI Agents for tasks such as email generation, coding, and data analysis. Deloitte predicts that 25% of companies using GenAI will deploy AI Agents by 2025, growing to 50% by 2027. Gartner predicts that by 2028, at least 15% of daily work decisions will be made autonomously by agent AI, while 33% of enterprise software applications will include agent AI.
● From Reactive to Proactive AI Agents
By 2025, AI Agents will transform from reactive assistants to proactive problem solvers. They will not just wait for instructions but predict needs, propose solutions, and take autonomous actions. For example, AI Agents can suggest optimal times for meetings, breaks, or tasks based on users' energy levels and productivity patterns.
● AI Agents and Hyper-personalization
Through generative AI integration, AI Agents can generate customized responses based on users' preferences, background, etc. For example, AI Agents in retail environments might predict user interest in new products based on browsing history, purchase patterns, and social media activity.
● Emotional Intelligence in AI Agents
AI Agents will develop higher emotional intelligence, better understanding and responding to human emotions, providing more humanized interaction experiences.
● Multimodal Capabilities in AI Agents
AI Agents will develop multimodal capabilities, integrating visual, voice, and text data to provide richer interaction experiences.
● More Advanced Multi-Agent Systems
As technology advances, more complex Multi-Agent Systems (MAS) are expected to emerge, capable of task allocation and collaborative problem-solving.
● AI Agent Building Frameworks
More AI Agent building frameworks are expected to emerge, making it easier for developers to create and deploy AI Agents.
● Increased Integration with IoT and Personal Devices
AI Agents are expected to integrate more closely with Internet of Things (IoT) and personal devices, providing more intelligent and personalized services.
● Ethical AI and Agent Decision Transparency
As demand for ethical AI increases, AI Agents' decision-making processes will become more transparent to win user trust.
● Enhanced Communication and Collaboration Tools
AI Agents are expected to provide more advanced communication and collaboration tools to improve work efficiency and team collaboration.
2. Potential Challenges
● Ethical Considerations
As AI systems gain decision-making authority in areas such as healthcare, finance, and law enforcement, ensuring these systems operate fairly, transparently, and safely becomes crucial. Unrestricted use of AI could exacerbate social biases, violate privacy, and cause unintended harm, undermining the progress it aims to achieve. Therefore, responsible AI development and deployment is not just about regulatory compliance; it's about fostering a culture of responsibility, accountability, and trust.
● Regulatory Issues
The diversity of AI applications and rapidly expanding user base requires regulators to provide guidance for this technology. AI safety, governance, and misuse are major issues facing policymakers. Accuracy, bias, privacy, intellectual property, and transparency are other key concerns. For example, the prevalence of bias is concerning when AI is used for sensitive decisions like recruitment or lending, and transparency faces challenges in decision-making. Governance must also address issues like data privacy and market power concentration.
● Technical Limitations
Technical risks include errors and failures as well as security issues, including the potential for automated cyber attacks. The autonomy of AI Agents also raises ethical questions about decision-making and responsibility, as well as socioeconomic risks surrounding potential job displacement and over-reliance and disempowerment.
AI Agents' future development will show progress in multiple aspects but will also be accompanied by ethical, regulatory, and technical challenges. Addressing these issues requires cooperation between policymakers, industry leaders, researchers, and civil society to ensure AI development both adheres to ethical principles and promotes human potential.
Conclusion
In the crypto space, the emergence of AI Agents is not just a technological breakthrough but a revolutionary attempt to combine artificial intelligence with decentralization. Although most current projects are in their early stages, they have clearly sparked a wave of experimentation and innovation in the crypto market, like a breeze rising from the ground, starting from the smallest beginnings. In the future, AI Agents will have unlimited possibilities.